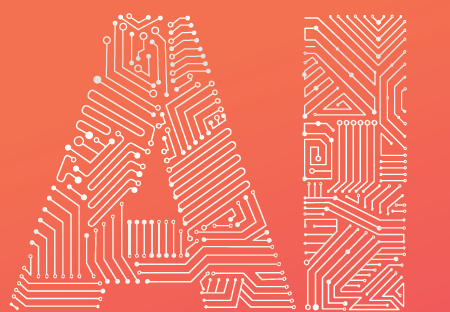
Over the last few years, technologies have emerged allowing enterprises to store all of their organizational data – in native formats – in what are known as large ‘Data Lakes’. This system was originally devised to provide a competitive advantage to the enterprise by enabling superior analytics on this comprehensive repository of raw and ungoverned data. However, because of the very fact the data is stored in different structures, without the benefit of optimized real-time data processing and discovery pipelines, the results have been disappointing. Data lake infrastructures have become as slow and stale as traditional business intelligence (BI) and data warehousing systems – which is what the enterprises were trying to get away from in the first place.
At the end of the day, big data is not just about having an infinite amount of data, it’s about having the right set of technologies and solutions to understand the patterns emerging from that data and gleaning actionable insights to help inform business decisions. Think of it like storing a supercar in a museum, you can admire it only for so long. If you can’t drive it and feel the power it provides, or use to it to get from point A to point B then all you have is a very expensive (albeit beautiful) piece of metal that can’t really do the job of a car.
Given the amount of data in storage and circulation today, it is actually impossible for a human or even a team of humans to parse all of this data in order to extract intelligence from it, especially at the pace at which data is being generated or ingested. Smart enterprises are now embracing technologies that provide data management and analytical capabilities combined – including artificial intelligence (AI) and machine learning – to understand these large and complex volumes of data. These systems put the power of AI into the hands of business users, who then with a few clicks and minimal development skills, can develop AI and machine learning models to swim through their enterprise data lakes to glean interesting insights from their data.
However, there are pitfalls to machine learning models in that the intelligence is only as good as the data that is being analyzed for insights. Having a foundational master data strategy with a focus on quality and governance is essential, but the key component is continuous improvement. Companies absolutely need a plan for continuously improving data quality based on “learned” insights – to yield the most from any enterprise data.
Some industry verticals have been quick to embrace the power of AI they and are ahead of most others. Foremost amongst them being finance and retail, especially those companies with a focus on the end consumer. With the growth of social media, a virtual treasure trove of consumer data about spending habits, life events, interests, grievances, likes & dislikes, social relationships and belief systems is available in the public domain. Mining this data can give the competitive edge to companies who want to target these consumers with their products and services or create a positive experience for them. Not surprisingly, a lot of the adoption of AI can be seen in the areas of sales and customer relations management, for use with targeted marketing and personalized recommendations. Here are some highlights from the field:
Retail: Curating personalized and automated content for end consumers
In today’s retail environment, consumers make purchase decisions based as much or more on the product reviews, specifications, and detailed descriptions available online as they do with physical contact with the product itself. It is paramount that products be discoverable and found, before the competitor’s products. The use of machine learning models that analyze past searches, buying patterns and demographic details, allows companies to target personalized content to individual shoppers. In addition, when displaying a product description to the potential shopper, product content can be adapted to match demographic and psychographic understanding in terms of language and culture, the nuancing of which will greatly enhance the level of personalization and the ultimate experience of the shopper.
This fundamentally changes the entire paradigm of content curation for retailers and merchandising teams who today commission survey after survey and deploy significant manual effort to understand how to target potential shoppers for their products.
Personalized recommendations
A shopper today, via search and rich product imagery, can come very close to finding products matching their tastes and preferences. But what if the retailer could display exactly the kind of product she would like to buy on the very top of the results? While she has a product open to view its specifications and description, what if she could see product recommendations she is much more likely to buy based on the insights gleaned from her past shopping history?
For every potential shopper, these recommendations can be different leading to trillions of suggested options and combinations. These simply cannot be created efficiently and dynamically without the power of AI and machine learning. Online retailers such as Amazon have been honing this technology for years and have now provided services for enterprises to use this power https://aws.amazon.com/mp/scenarios/bi/recommendation/
Natural language and conversational search
Companies such as The North Face have already piloted such search models on their website resulting in a 60% clickthrough rate and 75% total sales conversions for a sample size of 55,000 users. https://www.techemergence.com/artificial-intelligence-retail/
In short, data lakes are a mixed blessing. If used properly, with the right technologies, including data management solutions coupled with AI and machine learning, companies can capture highly useful insights and information from an asset they already possess – their data. If left alone, however, they turn to dataswamps – which as the term implies – are neither a source of actionable information nor a strategic asset.
I would really have to say that the more AI is being considered for IT sector job, the more people are likely to become unemployed. Let’s see what happens in the future to our jobs.
I recommend people to get into AI learning and development because the only scope it is the only scope left.
Very Informative. AI is the way to go forward for companies to excel.